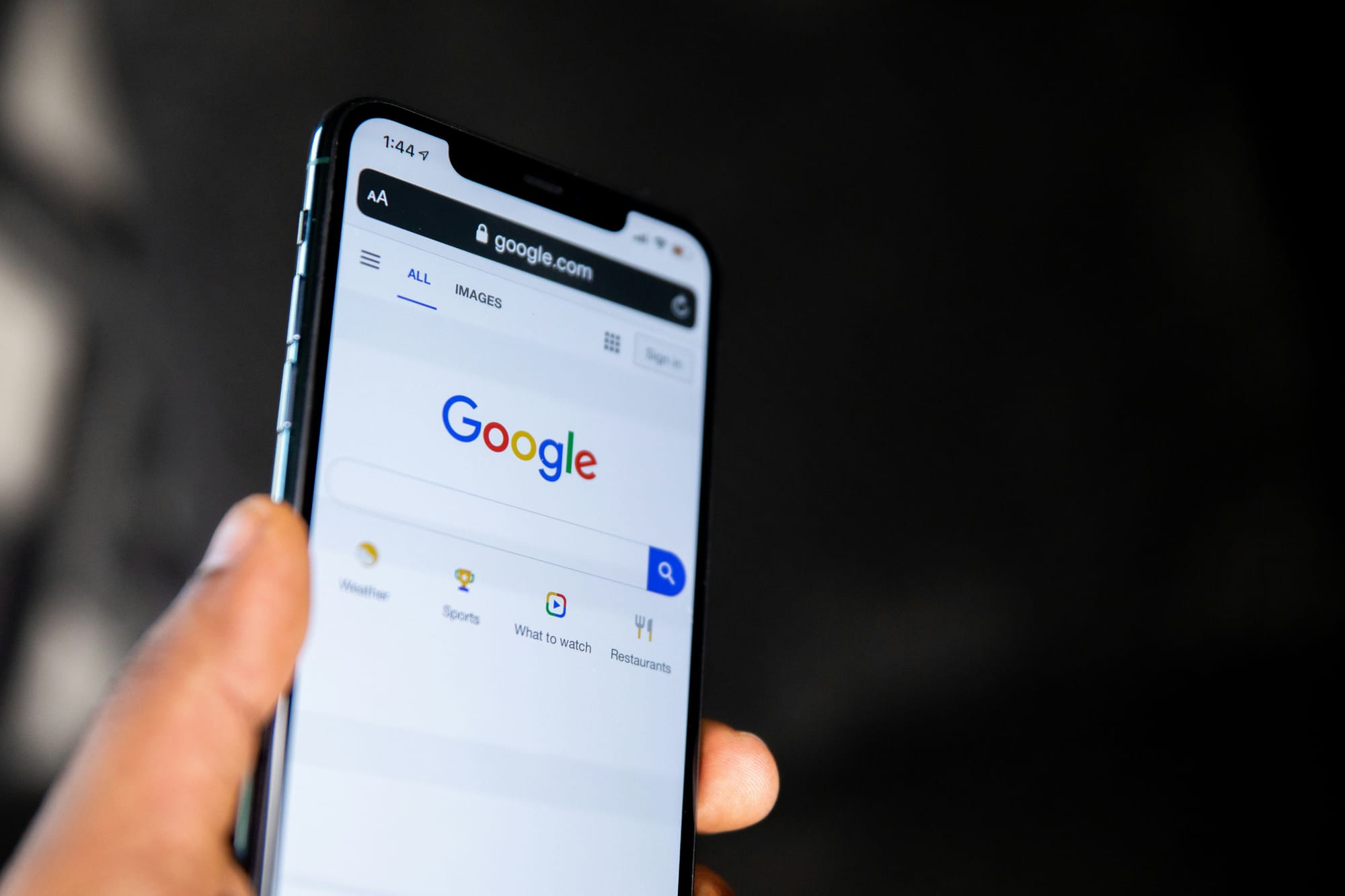
This is a column about AI. My boyfriend works at Anthropic. See my full ethics disclosure here.
I.
For more than a year now, I’ve had one eye trained on how generative AI will reshape the web. My primary fear has been that large language models like those found in ChatGPT are now good enough that large numbers of people are beginning to abandon traditional search engines, starving publishers and websites of the traffic and money they need to continue operating. While some publishers have made lucrative deals with AI labs, on balance the number of jobs in journalism is shrinking. And I’m not the only person worried: last month, Pew Research reported that about half of Americans believe that AI will be bad for journalism.
Until now, the effects of AI on the web have mostly been theoretical. But this week, an Apple executive testifying in the remedy phase of Google’s antitrust trial offered a piece of information that spooked observers of both companies.
Here are Emma Roth and Lauren Feiner at The Verge:
Google searches fell in Safari for the first time ever last month, Apple’s senior vice president of services, Eddy Cue, said during Google’s antitrust trial on Wednesday. “That has never happened in 22 years,” Cue added.
Cue linked the dip in searches to the growing use of AI, which Apple is now considering integrating into Safari. The rise of web search in AI tools like ChatGPT, Perplexity, Gemini, and Microsoft Copilot may make users less inclined to visit Google as their primary way of finding information.
The fallout from Cue’s revelation was swift: Google’s stock price declined 7.5 percent, erasing $150 billion or so of market value, before recovering 1.93 percent today. In response, Google hustled up a statement with the urgency of a flight attendant telling passengers not to panic during a particularly jarring moment of turbulence. The company said:
We continue to see overall query growth in Search. That includes an increase in total queries coming from Apple’s devices and platforms. More generally, as we enhance Search with new features, people are seeing that Google Search is more useful for more of their queries — and they’re accessing it for new things and in new ways, whether from browsers or the Google app, using their voice or Google Lens.
The first thing this statement does, quietly, is confirm that Cue’s testimony is accurate. (Something you can’t always take for granted with Apple executives these days.) The second thing it attempts to do is to reassure shareholders that it doesn’t matter. People might be making fewer searches in Safari, but they’re making more searches elsewhere. Google presents this as a natural evolution of search. You couldn’t always search by uploading a photo on your phone, for example; now you can using Lens, which is a feature of the Google app on iOS. If you love to search by taking photos, you might use the Google app more, and Safari a lot less.
I believe Google on this point. Rand Fishkin, an expert in search engine optimization and founder of the audience research company SparkToro, published a report last month that sought to put the rise of AI search in context against Google’s continued dominance. It found that Google searches rose 21.64 percent from 2023 to 2024. As Fishkin notes, this seems to be consistent with what Google CEO Sundar Pichai said when the company rolled out its AI overviews last year: that people who see them tend to do more searches.
Of course, the health of the web is not determined by the number of Google searches alone. Equally important is where people get their answers — and increasingly, they are getting their answers on Google. Multiple analyses have now found that Google’s AI overviews have resulted in declines of 70 to 80 percent in the click-through rates to the web pages from which they derive their information. That’s 70 to 80 percent fewer visits to web pages, and one of the primary web page-producing industries is shrinking accordingly: CNN, Vox Media, HuffPost, and NBC are among the publishers that have announced layoffs in 2025 so far.
If only to reassure its shareholders, Google would prefer to leave the story there: its search engine still legitimately ascendant, and the threat of disruption largely neutralized. (Disruption from challengers, at least. There’s still the risk of state-mandated disruption: the US government is currently seeking to force the company to spin out its Chrome browser and divest itself of core pieces of its advertising monopoly.)
But investors’ panic earlier this week was not entirely unwarranted. There are signs of an actual shift happening in search, and it is not to Google’s benefit.
It is not to the benefit of the web, either.
II.
One of the week’s best-read feature stories was written by James D. Walsh at New York Magazine. Its headline: Everyone is cheating their way through college.
It opens on Roy Lee, the Columbia University student who made headlines earlier this year by releasing software designed to help engineers cheat their way through technical interviews at tech companies. (He subsequently raised $5.3 million to build an app that helps you “cheat on everything”; we talked to him on Hard Fork, too.)
To Walsh, Lee is only the most visible example of an increasingly undeniable trend. “In January 2023, just two months after OpenAI launched ChatGPT, a survey of 1,000 college students found that nearly 90 percent of them had used the chatbot to help with homework assignments,” Walsh writes.
It has only accelerated since then; one study found that AI usage among college students increased from 66 percent last year to 92 percent this year. Professors who can no longer reliably tell AI-generated assignments from student-written ones speak to Walsh of an existential despair.
In Silicon Valley, this is what is known as product-market fit.
And this market, unlike search, is competitive: as Walsh notes, students don’t only use ChatGPT: they use Google’s Gemini, Anthropic's Claude, and Microsoft’s Copilot, among others.
For the moment, Google’s AI overviews seem to have quelled the possibility of a sudden mass defection away from its core search engine. But it’s now clear that for the first time in decades, a generation is growing up with the possibility of using something other than Google as its default search. Today, they’re using ChatGPT to do all their homework assignments. By the time they graduate, they may be using it to do almost everything else.
Google knows this, which is why it is seeking to pre-install Gemini on smartphones wherever it can. The company is having some success; Gemini is the second-most used chatbot after ChatGPT, according to Fishkin’s analysis, and Google overall handles 373 times as many searches as OpenAI’s bot.
But while there was once a time when the company could hand Apple $20 billion for default search placement and spend the rest of the year relaxing, Cue’s testimony shows why that is no longer the case. Bit by bit, current and future generations are shifting their habits away from traditional search and toward chatbots. Google can spend its many remaining billions of dollars on implementing Plan B. But it remains unclear what anyone else is supposed to do.
Elsewhere in the case: Mark Gurman argues persuasively that Cue's primary objective was to convince the judge that the search market is already so competitive that he should allow Google to continue paying it $20 billion a year. And M.G. Siegler has some more theories on why search declined on Safari.
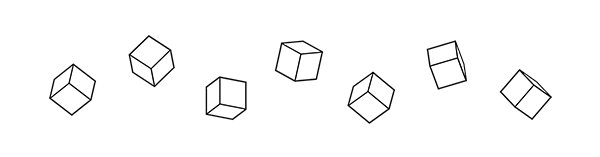
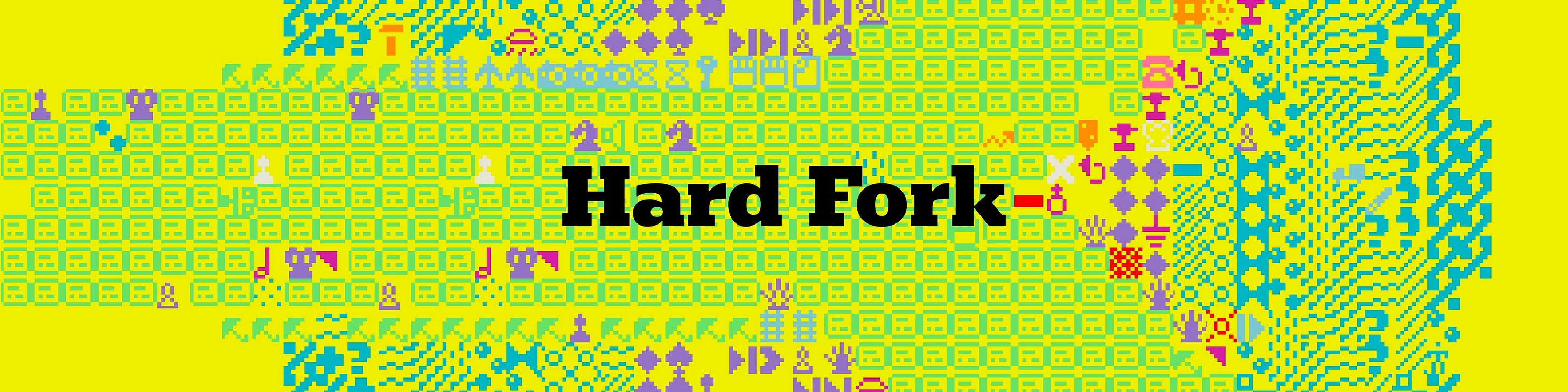
On the podcast this week: Kevin and I discuss how freedom came to the App Store, and Apple's disastrous loss in court last week. Then author Karen Hao stops by to discuss her new book Empire of AI and the downsides of pursuing massive scale. And finally, I introduce Kevin to the world of Italian brainrot.
Apple | Spotify | Stitcher | Amazon | Google | YouTube
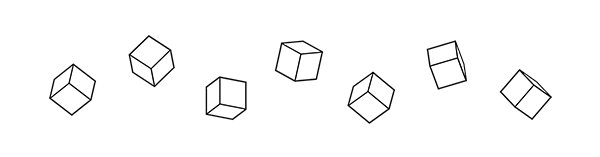
Sponsored
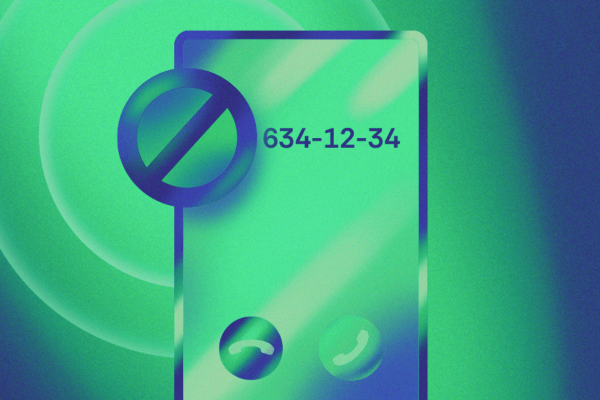
We’ve been trying to reach you...
…about your car’s extended warranty. Tired of getting those calls? Here’s your chance to protect yourself, your personal data, and your sanity. Incogni is a personal data removal service that scrubs your sensitive info (think: SSN, DOB, home addresses, health information, and contact details) from the web. With Incogni, you’ll worry way less about: identity theft health insurers raising your rates based on info from data brokers robo and spam calls scammers taking out loans in your name.
Protect your personal info + get 55% off your annual plan with code PLATFORMER
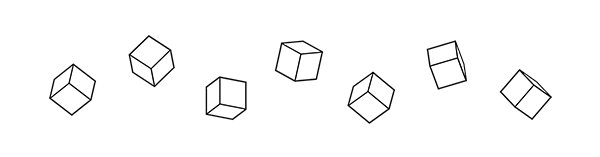
Governing
- The majority of Trump’s memecoin’s top holders are likely foreign, as many have used foreign exchanges that ban US users, this investigation showed. (Leonardo Nicoletti, Anthony Cormier and David Kocieniewski / Bloomberg)
- Democrats are calling for probes into Trump’s coins and its backers. (MacKenzie Sigalos / CNBC)
- Sam Altman said in Senate testimony today that it would be “disastrous” for the US if companies required government approval to release AI software, going back on his suggestion for regulation just two years ago. (Gerrit De Vynck and Nitasha Tiku / Washington Post)
- US embassies and the State Department have pushed countries negotiating tariff deals to approve US satellite companies including Starlink, documents show. (Jeff Stein and Hannah Natanson / Washington Post)
- The FDA has reportedly been meeting with OpenAI to discuss the agency’s use of AI in drug evaluations. (Zoë Schiffer, Emily Mullin, and Will Knight / Wired)
- Apple asked a court to pause an order that requires it to let App Store developers direct users to the web for in-app purchases without paying a commission. The chutzpah! (Josh Sisco and Mark Gurman / Bloomberg)
- The Trump administration reportedly plans to rescind some AI export controls on chips established under Biden. (Stephanie Lai and Mackenzie Hawkins / Bloomberg)
- Losing out on China’s estimated $50 billion potential AI market in the next few years would be a “tremendous loss,” Nvidia CEO Jensen Huang said. Particularly to Nvidia. (Ashley Capoot / CNBC)
- The CFPB will not prioritize enforcing a rule that classified buy-now-pay-later providers as credit card issuers, it said. (MacKenzie Sigalos / CNBC)
- It also discontinued its order to supervise Google Payments, reversing a Biden-era decision. (Paige Smith / Bloomberg)
- An appeals court rejected the FTC’s challenge to Microsoft’s $69 billion acquisition of Activision Blizzard. (Mike Scarcella / Reuters)
- Meta’s Oversight Board is looking at two cases involving videos that show teachers hitting children in school settings. (Oversight Board)
- Conservative activist Robby Starbuck sued Meta alleging that its Meta AI chatbot defamed him and spread false statements about him, including that he was part of the Jan. 6 insurrection. (Sarah Parvini / Associated Press)
- New Hampshire became the first state to pass a “Strategic Bitcoin Reserve” bill, according to Satoshi Action Fund founder Dennis Porter. (Daniel Kuhn / The Block)
- A look into Jesse Cunningham, an SEO specialist who claims to have made money from flooding Facebook with AI-generated images of things to trick older people. (Maggie Harrison Dupré / Futurism)
- Open-source project curl is overwhelmed by low-quality “AI slop” vulnerability reports, its lead developer said. (Kevin Purdy / Ars Technica)
- A visual look at how much AI is straining scarce water supplies. (Leonardo Nicoletti, Michelle Ma and Dina Bass / Bloomberg)
- An argument for why personal use of ChatGPT is not that bad for the environment. (Hannah Ritchie / Sustainability by numbers)
- The Wikimedia Foundation is challenging the UK’s online safety rules and raising concerns that they may enable “vandalism, disinformation, or abuse” on Wikipedia. (Jess Weatherbed / The Verge)
- The Irish finance ministry suggested an amendment to an EU proposal that would require big tech companies to check the legitimacy of advertisers before posting ads for financial services. (Paola Tamma, Barbara Moens and Jude Webber / Financial Times)
- Turkish authorities blocked the X account of Istanbul’s jailed opposition mayor, Ekrem Imamoglu. (Orla Guerin / BBC)
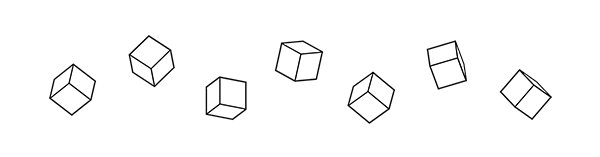
Industry
⭐ I talked with Grindr CEO George Arison for Fast Company. "We’re partly in the dating business, but we’re actually a social network," he told me. "So we don’t see dating fatigue here. What I do see is we need to do a much better job of making it easier for people who want to date to date. If there is one thing that people try other products for, it’s dating—and then they come back to Grindr." Click to learn more about the company's surprising new strategy, which includes declaring war on erectile dysfunction.
- OpenAI hired Instacart CEO Fidji Simo in a newly-created role as CEO of OpenAI applications, reporting to Sam Altman. (Shirin Ghaffary and Rachel Metz / Bloomberg)
- Simo will oversee sales, marketing and finance. (Mike Isaac / New York Times)
- OpenAI rolled out an initiative to help other countries build data center capacity for AI. (Shirin Ghaffary / Bloomberg)
- Anthropic is launching a new API that lets its Claude AI models search the web. (Kyle Wiggers / TechCrunch)
- Musk’s xAI will use Musk’s Tesla’s Megapack batteries for its “Colossus” supercomputer in Memphis. (Dana Hull / Bloomberg)
- TikTok told its advertisers that they’re confident in the future of the platform despite the looming US ban. (Sapna Maheshwari / New York Times)
- Meta is reportedly resuming efforts to build an AI-powered “super sensing” facial recognition feature for its devices, including the smart glasses. (Kalley Huang and Catherine Perloff / The Information)
- Meta appointed Robert Fergus, a co-founder of the Facebook AI Research lab, to lead its AI lab. (Riley Griffin / Bloomberg)
- Meta is reportedly in talks with crypto firms to introduce stablecoins to manage payouts. (Leo Schwartz and Ben Weiss / Fortune)
- Threads will begin testing video ads. (Sarah Perez / TechCrunch)
- Google is rolling out AI-powered tools and warnings to help users combat scams on Chrome. (Aisha Malik / TechCrunch)
- Amazon Web Services will spend $4 billion to build data centers and other cloud infrastructure in Chile. (Fabian Cambero / Reuters)
- Netflix will begin testing a TikTok-like vertical feed in its mobile app in the coming weeks. (Jay Peters / The Verge)
- Netflix debuted a new AI search tool that uses ChatGPT to give users a more conversational discovery experience. (Lauren Forristal / TechCrunch)
- Pinterest shares rose 15 percent after the company reported better-than-expected guidance in its first-quarter earnings. (Jonathan Vanian / CNBC)
- IBM CEO Arvind Krishna said the company has used AI agents to replace a couple hundred human resource workers and has hired more programmers and salespeople as a result. (Belle Lin / Wall Street Journal)
- Stripe unveiled new products, including a new AI foundation model for payments, stablecoin-powered accounts, and a “deeper partnership” with Nvidia. (Mary Ann Azevedo / TechCrunch)
- Match Group is laying off one in five managers as part of a 13 percent reduction in its workforce. (Natalie Lung / Bloomberg)
- Design company Figma announced AI-powered site and web app creation features. (Ivan Mehta / TechCrunch)
- The value of Bitcoin jumped above $100,000 for the first time since February. (Tanaya Macheel / CNBC)
- A look at how full autonomy of an AI agent remains a challenge despite agents already showing sophisticated decision-making capabilities. (Lucy Colback / Financial Times)
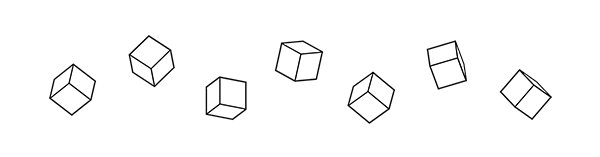
Those good posts
For more good posts every day, follow Casey’s Instagram stories.
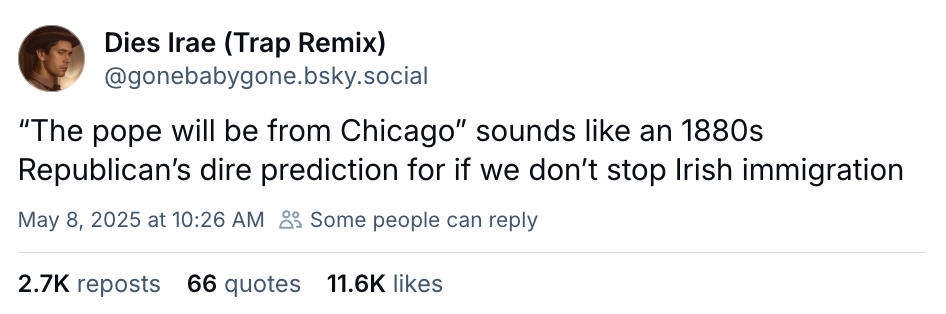
(Link)
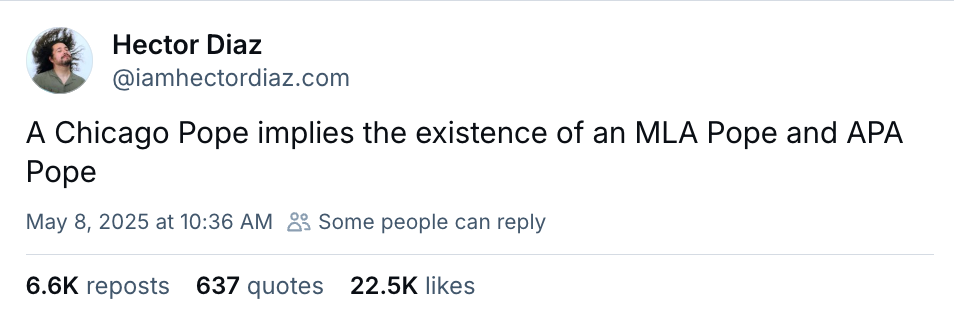
(Link)
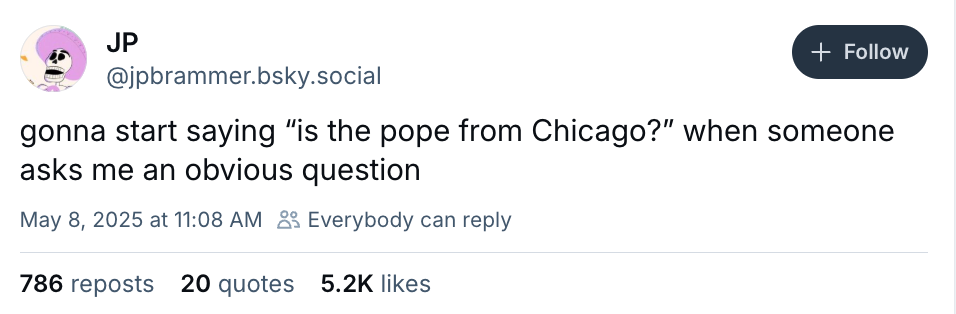
(Link)
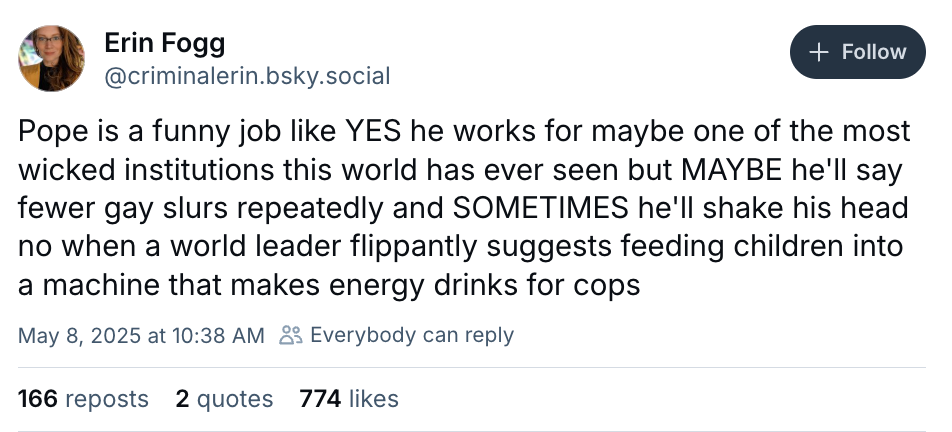
(Link)
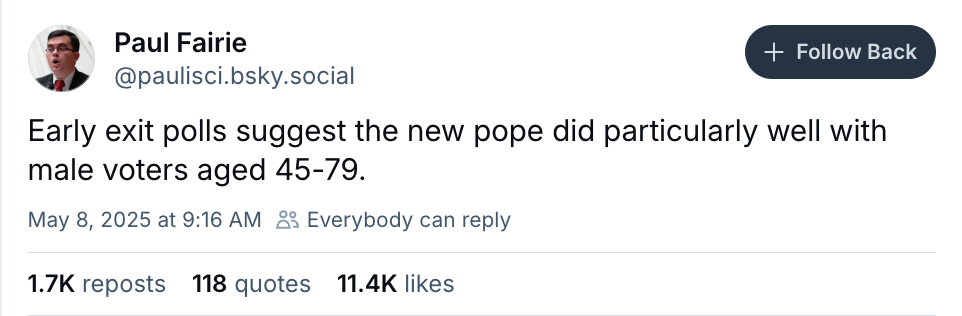
(Link)
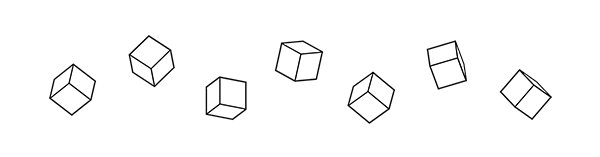
Talk to us
Send us tips, comments, questions, and Safari searches: casey@platformer.news. Read our ethics policy here.