A Philosophy of Happiness Through the Uncurated Life
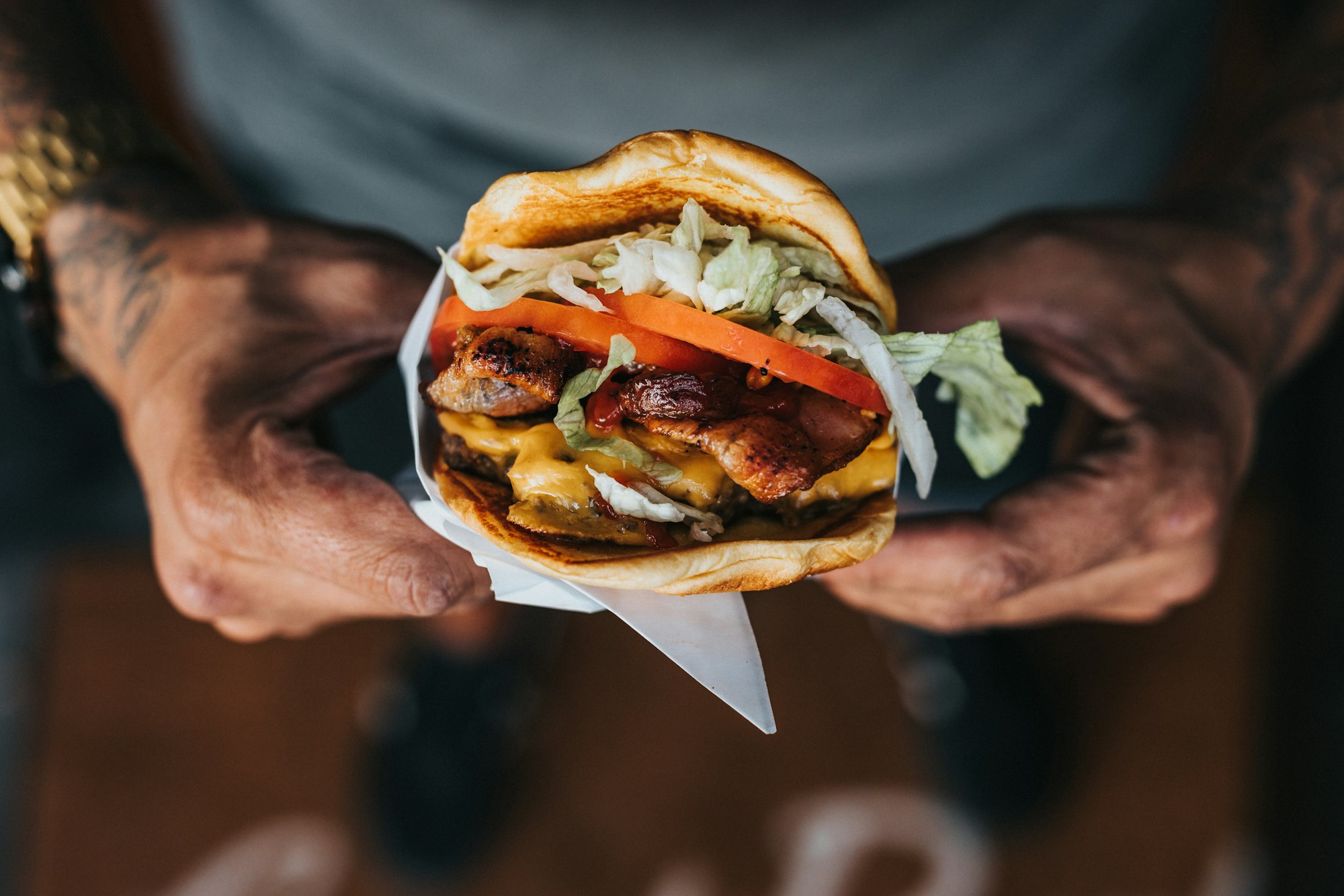
In 1953, Ernest Dichter—the father of motivational research—wrote that the American consumer was no longer purchasing soap to clean themselves, but to feel clean.
Advertising wasn’t selling products.
It was selling identity.
A bar of soap promised not just hygiene but moral worth.
Fast-forward seventy years, and you can trace a straight, greasy line from Dichter to Instagram meal-prep influencers, YouTubers waxing poetic about minimalism from $4,000 Herman Miller chairs, and Twitter productivity gurus who wake up at 4:30 a.m. to drink bulletproof coffee and document their sense of superiority.
We didn’t stop at soap. That instinct—to purchase meaning, to wear values like accessories—expanded until it swallowed almost everything. Now, even our meals, hobbies, outfits, and downtime are curated to project identity. Underneath it all is the same pitch Dichter uncovered: you are what you consume. Only now, it’s not just what you buy—it’s how you present it. Life itself is packaged, stylized, and sold back to the self.
Jean Baudrillard summed it up:
“We are at the point where consumption is laying hold of the whole of life, where all activities are sequenced in the same combinatorial mode, where the course of satisfaction is outlined in advance, hour by hour, where the ‘environment’ is total—fully air-conditioned, organized, culturalized. The beneficiary of the consumer miracle also sets in place a whole array of sham objects, of characteristic signs of happiness, and then waits (wars desperately a moralist would say) for that happiness to alight.”
We’re miserable.
Aren’t we?
Lonelier, more anxious, more frustrated, and more exhausted than ever.
Not because we lack comfort or tools or access—but because we’ve staged too much of ourselves.
We’ve turned living into editing. When every bite is a performance, every outfit a brand decision, every hobby a pitch, there’s no space left for boredom. Or rest. Or actual pleasure. We scroll through each other’s highlight reels while quietly assembling our own, haunted by the suspicion that everyone else is doing it better—and forgetting to live in any of it.
I crashed hard after the pandemic. It wasn't cinematic, and it wasn't a breakthrough. It was dull. Exhausting. Slow. It was a kind of rot, spreading inward. At first, I thought I was just tired. Overstimulated, under-rested, another victim of collective burnout. But it got worse. My routines—ones I’d once clung to like scaffolding—started to feel grotesque. Wake up, hydrate, gratitude journal, morning sunlight, stretch, optimize. For what? I couldn’t answer. I didn’t even want to ask anymore. I’d spent years trying to build a life that looked good on paper, sounded smart in conversation, and played well on social media. I had all the systems, all the trackers, all the polished, adult routines. I was a walking Notion template. And...I was hollowed out. I wasn’t living—I was managing myself like a brand asset. I didn’t know how to stop.
What broke me wasn’t a single moment. It was the accumulation of hundreds of tiny ones that didn’t feel like living. Eating meals I didn’t enjoy but felt obligated to consume because they were “clean.” Turning walks into podcasts into productivity. Posting through loneliness and calling it community. Trying to be impressive while privately falling apart. I felt like a parody of myself—curated, competent, and completely numb.
There’s a Camus quote I keep coming back to:
“At any street corner, the feeling of absurdity can strike any man in the face. As it is, in its distressing nudity, in its light without effulgence, it is elusive. But that very difficulty deserves reflection. It is probably true that a man remains forever unknown to us and that there is in him something irreducible that escapes us.”
Absurdity is precisely the word.
One night, I just sat on the floor and thought: none of this is working. None of this is helping. I’ve done everything modern culture told me to do to be happy, successful, fulfilled. I was tracking everything but feeling nothing. That was the moment something cracked open in a quiet, defeated realization: I don’t want to live like this. I don’t want my life to be a performance. I don’t want to be optimized. I just want to feel human again. I want to be messy. Boring. Unimpressive. Real.
In the months, years since the pandemic's peak, I've been unable to reconcile the cognitive dissonance. Seeing the inauthenticity and performance of modern happiness has made it impossible to achieve happiness through the same means. There's a falseness to it all, a sense of how fragile the facade actually is.
After the collapse, after the burnout, after the creeping dread that none of the things I’d been told to care about were making me feel human, I started noticing what actually felt good. Not "aspirational" good. Not "productive" good. Just good. A grilled cheese sandwich eaten in the sun. A day without notifications. Saying no and not explaining. I didn’t see it as a philosophy. I just knew I felt less fake. Less hollow. Less like I was performing a version of myself I couldn’t stand anymore. Over time, I started tracing a pattern. What if I stopped managing my life like a brand? What if I let it be messy, private, low-stakes? What if that was enough?
The Ordinary Sacred: A Philosophy of Uncurated Life
The Ordinary Sacred is my idea for a philosophy of presence without spectacle. A life without audience. A refusal to curate the self into something consumable. It honors sufficiency over scale, texture over narrative, and experience over optics. It says: the real, unpolished, unposted life is enough—and always was.
It names the thing without dressing it up. It doesn’t try to win attention. It doesn’t demand belief. It simply offers a shift—a posture of attention, a refusal to perform, a commitment to being here. And if it sounds pretentious, hell - you should see the rest of my blog.
The Ordinary Sacred turns directly into the mundane. This is where meaning lives. In unremarkable afternoons. In laughter that goes unrecorded. In friendships that don’t need captions. In lives that never go viral. In meals that don’t “flush toxins” or whatever absurd promise is making the wellness rounds this week.
This is the closest I’ve come to a working, personal theory of happiness. Not the performative kind. Not the kind with a morning routine and a five-year plan. Not the kind you can monetize, or coach, or convert into bullet points or turn into a cult. The kind you feel in your teeth when you bite into something good, or in your chest when you laugh too hard, or in your shoulders when you realize—for the first time all day—that you’re not clenching.
It starts with a full-body, bone-deep refusal. To stop turning your life into content. To stop polishing your edges so you can be easier to consume. To stop translating every moment into something brand-safe, clever, legible. To stop acting like joy only counts if it comes with a graph and performs well in metrics.
We live under systems—economic, cultural, digital—that demand we strive to be impressive. Inspirational. Aspirational. Permanently visible. Permanently performing. Eternally, achingly unsatisfied. We’re trained to ask, before doing anything: Will this make good content? Will this signal something useful? Will this get me closer to who I’m “supposed” to be?
The Ordinary Sacred says: fuck all that. Be ordinary. Be quiet. Be offline. Do things because they feel good, or because they’re funny, or because they’re yours. Not because they’ll look good later. What's most valuable isn't what's exceptional, curated, or performative, but rather what's common, authentic, and directly experienced.
It’s not anti-tech, or anti-ambition, or a cry to return to the earth from whence, etc. You can still use GPS. You can still have a favorite brand of headphones. You don’t have to churn butter or reject civilization or free float into the void. You just have to stop selling yourself to yourself.
It’s lo-fi, low-stakes, non-viral. It’s fully alive. It gives you permission to breathe. To be boring. To be happy in a way that no one else gets to gatekeep or approve.
TLDR: The Ordinary Sacred:
There are no habits, wellness tips or life hacks here. Just solid, practical and deeply personal decisions in a culture that pushes all of us to perform.
They’re not the whole picture.
Only the first four that made sense.
- Eat for yourself, not for anyone else — Real food, real joy.
- Work to pay your bills, not to validate your worth — Labor is not identity.
- Buy things, not signals — Use and want over performance.
- Live your own life, not for your feed — Escape is freedom.
Eat For Yourself
Wellness influencers parade turmeric like it’s a gift from an alien super being. Kale is fetishized. Everything is raw, anti-oxidized, adaptogenic. Food is now a purification ritual for people afraid of living in their bodies. It’s asceticism disguised as luxury. We pretend a spirulina bowl is satisfying. It’s fucking not. It tastes like damp grass and self-denial.
Food has become a moral minefield. We talk about "clean eating" like impurity is contagious. We moralize portions. We collapse nutrition into discipline, and discipline into righteousness. It’s no longer just a question of what you eat—it’s a referendum on who you are. Hungry? Control it. Ate something with cheese? Better confess. Craving carbs? Repent.
We count calories like sins. We call dessert a "guilty pleasure." We look at our plates and think about punishment. All of it delivered in a glossy wrapper of faux empowerment—"wellness" that is just diet culture with a better font.
And this grift hits hardest where it always does: at the intersection of class, image, and shame. The wellness industry sells restriction as virtue, but only if it comes in the right package. Smoothie bowls cost $14. Alkaline water is aspirational. Fasting is chic if you’re thin and rich, disordered if you’re poor and fat. We have created an entire language of virtue and failure out of what people put in their mouths. And we judge. Constantly.
It's not health. It's a caste system built on quinoa and quiet cruelty.
Food is not fuel. It’s food. It’s greasy, salty, sweet, hot, cold, cheap, satisfying. It’s a double cheeseburger from a place with flickering lights. It’s fries at midnight, cereal for dinner, gas station snacks on a road trip. It’s eating something because it smells good, not because it fits your macros or matches your aesthetic. It doesn’t need to be “activated.” It doesn't need to be spiritualized. It doesn't need to be posted.
Eat the thing. Eat it hot, with your hands, juice on your chin. Don’t wait for the right angle. Don’t explain it. Don’t count it. Taste it.
And when you taste it—really taste it—you’ll understand why Brillat-Savarin, the French epicure and proto-food philosopher, opened his Physiology of Taste with this line: “Tell me what you eat, and I will tell you what you are.” He wasn’t moralizing. He was celebrating. He argued that pleasure from food was not only real, but necessary: “The pleasures of the table belong to all ages, to all conditions, to all countries, and to all areas; they mix with all other pleasures and remain at last to console us for their loss.”
Food isn’t a weakness. It’s a foundation. It outlives beauty, relevance, even sex. It is the last pleasure to go. And still, we punish ourselves. We eat alone and scroll silently past staged plates that make us feel worse. We are told to crave less, shrink more, fast longer.
And we carry that punishment into our bodies. The obsession with weight has become its own religion—worship of thinness masquerading as discipline, devotion measured in deprivation. Your worth reduced to numbers: grams, inches, pounds. Your character tied to how well you say no to hunger. This isn’t health. This is control.
Eat with others. Eat things that drip. Let food be messy and close and real. As M.F.K. Fisher wrote in 1943, during wartime rationing no less, “Sharing food with another human being is an intimate act that should not be indulged in lightly.” That intimacy—the kind born from greasy hands passing a burger across a table—is more nourishing than any supplement, any powder, any smug, made-up-toxin-free pile of performative buckwheat.
Seneca, even in his Stoicism, admitted this: “We should look for someone to eat and drink with before looking for something to eat and drink.” Eating alone isn’t just lonely. It’s unnatural.
A cheeseburger—greasy, simple, immediate—is not a compromise. It’s an honest answer. You don’t have to justify it. You just have to chew.
Simple happiness honors appetite. It mocks dietary performance art. It sees virtue signaling in quinoa bowls and says: you are allowed to want salt. You are allowed to want fat. You are allowed to be full. You are allowed to be more than full. You are allowed to enjoy yourself and your self.
The world doesn’t need more photographed smoothie bowls. It needs more loud laughter over shared fries, more sauce on shirts, more meals that begin with hunger and end in satisfaction, not shame. That’s living. That’s the ethic.
This—this mess, this unfiltered joy—is part of what The Ordinary Sacred defends. A return to trust. In our bodies. In our appetites. What we eat is not a performance. It’s a reunion with our selves, with each other, with the parts of life we’ve been taught to edit out.
Work to Pay Your Bills
The modern economy is a pantomime of purpose. "Do what you love," we are told. As if love is scalable. As if passion is billable. As if your deepest sense of meaning should report to a quarterly KPI.
It sounds harmless, even inspiring, until you realize it’s a trap. Once you collapse identity into labor, every moment becomes a performance review. You’re not just working. You’re branding. You’re optimizing. You’re pretending that the thing you’re doing for rent is your soul’s calling. And if it isn’t? Then you’ve failed—not just professionally, but existentially.
There’s something uniquely exhausting about being alive in an era where your job description is expected to double as your identity. You’re not a coder—you’re a Ninja, a Guru, an Evangelist. The Ordinary Sacred looks at all that and laughs. No one needs your LinkedIn headline to be poetic. Just make rent. Go home. Be a person.
Work is work. You show up. You do the thing. You clock out. That doesn’t make you lazy. That makes you sane.
And yes, there’s dignity in labor. But there’s also dignity in limits. Working more doesn’t make you more if it doesn’t make you more money to support your family, to live your life. You can be brilliant and not be busy. You can be wise and take naps. You can be proud, virtuous (if that's your schtick), and completely unscalable.
There's comfort here in Aristotle. In Politics, he writes, “the end of labor is to gain leisure,” and defines leisure not as rest from exhaustion, but as the space in which real life begins. It’s attention. It’s spaciousness. It’s where we cultivate thought, relationships, and the kinds of things you can’t track on a company dashboard. But it’s also binge-watching a show until your brain goes blank because, fuck it, that’s what you want to do, sitting on the couch at the end of the day with the person you love more than anyone else in the whole damn world and cycling through episodes of Star Trek. Because that’s where the happiness is.
Seneca picked up that thread centuries after Aristotle. In his essay On the Shortness of Life, he observed, “It is not that we have a short time to live, but that we waste a lot of it… Life is long if you know how to use it.” He was writing to Romans who were overcommitted, overextended, and distracted by ambition. Sound familiar? His solution wasn’t to do more. It was to stop mistaking busyness for worth.
In his 1932 essay In Praise of Idleness, Bertrand Russell argued that the modern worship of work had become a sickness. “The morality of work,” he wrote, “is the morality of slaves, and the modern world has no need of slavery.” What he proposed wasn’t laziness—it was balance. He imagined a world where people worked less not because they lacked ambition, but because they had other things worth doing: reading, thinking, walking, being. His version of a good life wasn’t crammed with productivity. It was spacious enough for thought, curiosity, and actual rest. Work wasn’t the point. Living was.
But here we are—half-human, half-product, refreshing inboxes and waiting for the dopamine of a Slack ping. Watching people post hustle reels while secretly googling burnout symptoms.
Stop making your job your personality. Pay your bills. Turn off your phone. Leave the spreadsheet in the cloud and go make dinner. You are not a commodity. You are not your engagement metrics. You are not your title or your side hustle or your carefully sharpened personal brand.
You are a person. Work enough to sustain that. Then stop.
Buy Things, Not Signals
There are whole industries now that don’t sell products—they sell proof. Proof that you’re tasteful. Proof that you’re refined. Proof that your life is intentional, curated, and on trend. There are couches you can’t nap on, plates you don’t eat off, clothes you only wear to signal restraint. These things don’t serve life. They stage it.
We’ve convinced ourselves that taste is the same thing as virtue. That a beautiful apartment is a moral accomplishment. That buying the right lamp means you’ve achieved some kind of internal alignment. But what’s really happening is this: we’re buying our own dissatisfaction. We’re furnishing for the feed. We’re building rooms we don’t relax in. Can’t relax in. Can’t even live in.
Curation culture is a profound narrowing. It's conformity with better lighting. You are allowed to be unique, but only in ways the algorithm understands: neutral tones, soft textures, minimalist aesthetics with maximum expense. Your life becomes a set. And you? A prop.
The Ordinary Sacred doesn’t care what your living room looks like on camera. It doesn’t care if you have matching decanters or poured concrete bookends. It asks: is your life usable? Do your things serve you, or do you serve them?
This tension isn’t new. The Stoics wrote about it constantly—not to glorify suffering, but to keep the focus where it belonged. Musonius Rufus, in his Lectures, writes:
“We must not admire those who own great possessions, but those who have the strength to do without them. For it is not he who has little, but he who desires more, that is poor. The man who is not in need is not the one who has much, but the one who can go without much.”
That’s not minimalism-as-aesthetic. That’s minimalism as escape from the mental leash of consumer anxiety. From the constant itch of want. From the cultural myth that more—prettier, sleeker, more tasteful—is the same as better.
Socrates, when walking through the Athenian marketplace, is reported to have said, “How many things there are in this world of which I have no need.” Imagine saying that in a shopping mall. Imagine feeling it in your bones while scrolling through influencer home tours. It’s not asceticism. It’s relief.
Aim lower. Buy what works. Buy what brings you real joy, not curated aspiration. You don’t need a capsule wardrobe. You need pants that don’t dig into your ribs when you sit. You don’t need artisanal Japanese storage boxes. You need to be able to find your damn keys.
This is not an attack on beauty. Beauty matters. But performance is a parasite that feeds on it. When the primary function of an object becomes its optics, it’s already dead. A beautiful object that demands anxiety isn’t beautiful. It’s a burden.
There is no moral prize for restraint. No award for matching the algorithm’s idea of dignity. Buy dumb stuff. Buy ugly mugs that feel good in your hand. Buy cozy blankets in loud colors. Buy the thing that reminds you of your childhood, even if it’s kitsch. Let your home look like you actually live in it.
Do it for you. Not for the performance.
Live Your Own Life
This is the big one. This is the hardest one. Because even if you eat what you want, and work to live, and buy things that serve you, there’s still the temptation and the judgement of the performance. The hum. The constant background noise of the self being watched.
And worse: the constant comparison.
You check your reflection in the café window. But you’re not really looking at yourself. You’re comparing that reflection to someone else’s post. Someone else’s morning. Someone else’s outfit, hair, discipline, joy. You half-laugh and wonder how it would’ve looked on camera. You draft the caption for the moment before the moment has even ended. You narrate your own life in real time, for an imaginary audience who may or may not ever see it. Every good day is content-in-waiting. Every bad day is a potential comeback arc. We have internalized the lens. And through that lens, we are constantly measuring.
Their kitchen is cleaner. Their life is quieter. Their goals are clearer. Their grief is more graceful. Their version of authenticity looks better than yours feels.
Social media didn’t create this instinct—it just strapped a monetization engine to it. Humans have always sought approval. But now we seek it in obsessively, constantly, publicly, and with analytics. Everything becomes a pitch. Even our sincerity starts to feel rehearsed. Erich From, in The Sane Society, warned of the rise of what he called the “marketing orientation,” where a person’s value is determined by their ability to sell themselves. “The self is experienced as a commodity,” he wrote, “whose value and meaning are extrinsic to the self and lie in its exchangeability.”
And what is Instagram but an exchange market for identity? What is TikTok but a derivatives market for personality? We are priced by our virality, our reach, our relevance. Every scroll becomes a series of self interrogations: Who’s doing life better? Who’s further along? Who’s winning?
Get out. Get the fuck out. Not forever. Not in dramatic fashion. Just enough to find your pulse again. Just long enough to remember that you were never supposed to be measured against everyone else’s highlight reel.
Go for a walk and don’t track your steps. Sit in a room without documenting the lighting. Tell a joke that doesn’t get recorded, doesn’t get retweets, doesn’t get remembered by anyone but the person you told it to. Laugh in a way that’s too loud. Be unflattering. Be unreadable. Wear the weird shirt. Take the ugly photo and don’t post it.
I’m not flogging the dead-horse of "authenticity." That word has been bled dry by marketers. Everything is "authentic" now—packaged transparency, curated vulnerability, aesthetic relatability. What we’re talking about is something rarer: being invisible. Not erased. Not ashamed. Just free from the eyes that don’t matter.
There is a kind of peace in the unrecorded life. A soft, slow quiet that doesn’t ask you to perform. Montaigne understood this long before the internet existed. "I want to be seen as I am," he wrote, "neither better nor worse." And yet, he also kept most of his thoughts private. “The greatest thing in the world is to know how to belong to oneself.”
Be unrecorded. Be untagged. Be.
Marcus Aurelius, writing to himself in Meditations, saw the trap of reputation clearly. “Waste no more time arguing what a good man should be. Be one.” Be. And later: “Do not waste what remains of your life in speculating about your neighbors... how he did this or that, or what he said, or thought, or schemed. Look instead to what you have to do yourself.”
And Epictetus was even blunter: “If you want to improve, be content to be thought foolish and stupid.” It’s the opposite of branding. It’s the rejection of legibility. Improvement, he suggests, is internal. The rest is noise.
In Being and Time, Heidegger argued that modern life alienates us from authentic existence by pushing us into the “they-self”—a mode of being where we exist primarily through the eyes of others. We become anonymous, absorbed in what "they" do, think, expect. "Everyone is the other, and no one is himself," he writes. Social media has weaponized the they-self. And we volunteered.
You can undo that. Not with slogans. With practice. With attention. You can ask: who are you when you’re not being watched? Who are you when nothing is being documented? Who are you without the mirror of other people’s reactions?
You do not need to be legible. You do not need to be a narrative. You do not need to be consumed. You need to be present.
Attention is sacred. Give it to your life—not to the algorithm. And for the love of your peace: stop measuring. You were never supposed to be someone else’s content. And they were never meant to be yours.
The Counterarguments
Isn’t this just another aesthetic? Isn’t this just a different kind of performance—anti-gloss as gloss, curated messiness, low-res virtue signaling? Isn’t it just normcore for burnout millennials?
That’s the risk. Absolutely. Every idea can be flattened into content, ironized into branding, merchandised into a lifestyle product with a logo and a tagline. But that doesn’t mean the idea is empty. It means it’s vulnerable. It means you have to guard it.
In The Society of the Spectacle, Guy Debord warned that “everything that was directly lived has moved away into a representation.” Experience becomes image. Life becomes theatre. Even revolt becomes performance. “The spectacle,” he wrote, “is not a collection of images; it is a social relation among people, mediated by images.”
Which means that anything—including this—can be eaten by the spectacle and turned into another flavor of capitalism. That doesn’t make it meaningless. And it doesn't make it any less precious.
Living the Ordinary Sacred doesn’t mean you disappear. It doesn’t mean you never post again, or live in a cabin without Wi-Fi, or become the annoying guy at parties who lectures people about Instagram. It means you post less, and later, and without expectation. You don’t stage your joy. You don’t curate your dinner. You don’t turn every genuine thing into a teaser for your personality.
You let some things remain yours. Not in the sense of ownership. In the sense of privacy. In the sense that no one else gets to consume or be consumed by them.
Montaigne knew it, even in the 16th century. He wrote, “A man must keep a little back-shop, all his own, wherein to be himself, without reserve.” Not everything goes in the window. Not everything is for show. “If we have been able to live well and think well,” he continued, “we have lived enough.”
You are allowed to have a self that isn’t publicly vetted. You are allowed to experience a moment that doesn’t become story. You are allowed to live outside the logic of performance.
The Ordinary Sacred is a practice. And like any practice worth doing, it requires restraint. You resist the urge to narrate, to beautify, to stage. Not because those things are evil, but because they’re constant—and you need space to hear yourself think.
You can still enjoy the internet. You can still have fun online. But the minute your real life starts feeling like a draft folder for future posts, something is broken. The Ordinary Sacred is the attempt to stop that collapse. To create space between the event and the upload. To protect the sacred ordinary from the economy of spectacle.
This isn’t countercultural for the sake of it. It’s countercultural because the culture is sick. You don’t need to reject technology. You need to reject the demand to turn your soul into a feed.
Keep something for yourself. Leave something unposted. Let your life be bigger than your screen.
I'm not claiming this is a new idea. It draws from a lineage of thinkers and traditions that insisted the sacred was never elsewhere. It was always in the here and now—if you knew how to look.
Zen Buddhism and Stoicism give us the clearest gesture: chop wood, carry water. Enlightenment isn’t found in escape or spectacle. It’s found in full presence within the ordinary. The practice is the life. The life is the practice.
Phenomenology, especially in the work of Merleau-Ponty, roots all meaning in lived experience. There is no truth apart from the body, from perception, from being-in-the-world. The ordinary isn’t something to transcend. It’s the only ground we’ve ever had.
The Transcendentalists—Emerson, Thoreau, Fuller—understood that what’s called "divine" doesn’t live above us. It lives around us. Underfoot. In trees and rivers and silence and solitude. Thoreau wrote: “I went to the woods because I wished to live deliberately… and see if I could not learn what it had to teach.” He didn’t find truth in institutions. He found it in the texture of everyday life.
Thomas Moore, in Care of the Soul, wrote about tending to the world, not overcoming it. He reframed the sacred as something found in dishes, conversations, melancholy, repetition. Not transcendence. Depth.
Annie Dillard, in Pilgrim at Tinker Creek, knew a kind of fierce noticing. She found the infinite in the specific. The sacred in the granular. “How we spend our days is, of course, how we spend our lives,” she wrote. No spectacle. Just close, attentive being.
Where This Leaves Me
I got here the long way around. Through collapse. Through burnout. Through the slow erosion of energy, interest, and self. Through all the self-improvement and content and aesthetics and hustle and intention and systems and routines—until one day I just couldn’t do it anymore.
I kept looking for something that would make my life feel like mine. Something to click. Something to unlock the sense that I was really in it. Instead, I just got more polished. More watchable. More brandable. More tired.
I thought quitting would look like failure. But it didn’t. It looked like sitting on the floor and breathing. It looked like making scrambled eggs without narrating it. It looked like walking without tracking. It looked like being a person instead of a project.
There was no revelation. Just the quiet repetition of No.
No, I’m not posting that. No, I don’t care if this aligns with my "voice." No, I'm not tracking this. No, I’m not optimizing this meal, this moment, this body, this day.
And then—without realizing it—I was living differently. Still online. Still in the world. Just… off the feed. Out of the frame. Inside something I hadn’t felt in a long time: my own life.
That’s what The Ordinary Sacred is. You can't buy it. You can't sell it. It’s not an app. It’s not a coaching program. It’s not a proprietary system.
It’s just a way to live without submitting your life for approval.
It’s the dignity of being a person.
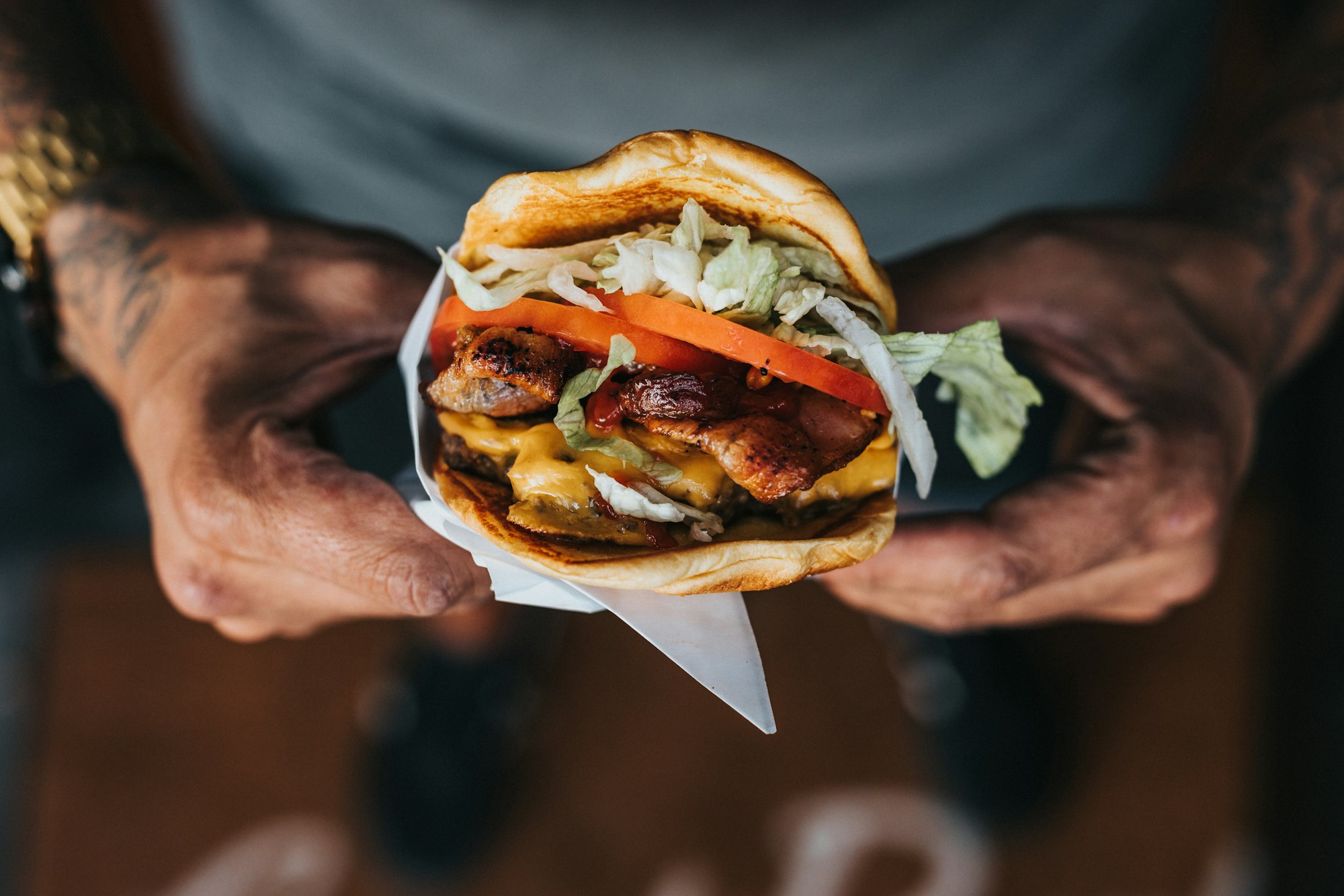