In the early 1980s, if you wanted posters to advertise a yard sale or a party, your options were pretty limited.
You could get some blank sheets of paper and some crayons or felt markers for the whole do-it-yourself experience, which was cheap but unless you were a professional illustrator the results were bound to leave something to be desired, if not be downright embarrassing. Otherwise you could get the local printer to do it but it wasn’t going to be very cost-effective, especially in such a small quantity. Each poster could end up running you the same price as a Big Mac – or even a whole Meal Deal – and with that kind of overhead it could make the whole yard sale endeavour smell much less appetizing. And so, usually it was back to the felt markers or whatever else you could find lying around the house, and being the tightwad you were you would not-so-happily contribute an additional indignity to the ever-growing mountain of your own personal shame, because you wanted that Big Mac for yourself, dammit.
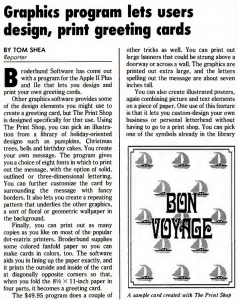
And let’s not even get started on greeting cards, those vile instruments of larceny that existed only to relieve you of your Big Mac money because if you didn’t buy them for every birthday, wedding, anniversary and holiday to give to every family member, friend, co-worker and all the other people you were even remotely acquainted with, you were certain to be outed as the tightwad you were and they might not want to be seen in public with you anymore. Which would leave you sitting in McDonald’s eating your Big Mac alone, plotting your revenge against Hallmark for engineering the single greatest extortion racket in the history of rackets. Curse you, Hallmark. Curse you!
But luckily for you, things weren’t going to remain this way forever. Home computer manufacturers began to get into some pretty serious price wars with each other, making their products much cheaper than they had been, and hence more accessible for tightwads like yourself. If you wanted to be really frugal, you could save your Big Mac money and convince your boss or parent to buy one! Totally win-win, right?
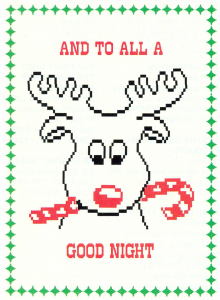
However, while the computer could theoretically handle the artistic part of things better than your own two thoroughly uncultured hands, there wasn’t yet e-mail or Facebook with which to send your digital creations, and there were no kiosks at Officeworks or Kinko’s where you could actually render your new digital servant’s creations to paper without having to pay that blasted printer all of your Big Mac money! You needed your own printing device (also co-incidentally called a printer) but you had to be patient because all you (or your parents or boss) could afford was a ‘daisy wheel’ model that punched out each character fully-formed like a typewriter, and unless you were proficient in ASCII art was quite useless for making posters and/or greeting cards, thus doing nothing to fill your stomach with more Big Macs. Curse you, Daisy!
But happily the printer manufacturers began to race for the bottom of the market (e.g. you) and soon dot-matrix models (which could print graphics) began to appear at a price more palatable to you (or whoever else you could talk into buying one). But then it turned out that designing the computer graphics yourself was tedious and time consuming, and you started longing for the felt markers and crayons. Now what?
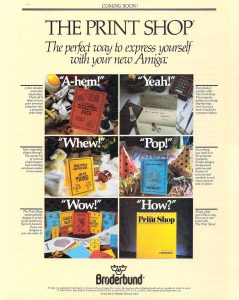
What you needed was software specifically designed to create posters and greeting cards without needing much effort on your part – a kind of… well, a computerised ‘print shop’ that wasn’t out to steal your Big Macs. Fortunately for you, a few enterprising individuals must have heard your pleas, because lo and behold, in 1984 software publisher Brøderbund released… well, ‘The Print Shop’, a program designed to create posters, greeting cards and other things without much effort on your part. If you had an Apple II that is. But you didn’t, because you were a tightwad who bought a Commodore 64 (or convinced someone else to) and so you had to wait an extra year before it came out for it (and the Atari 8-bit machines, also popular with tightwads after Jack Tramiel took Atari over and got into a price war with his former company). But you were happy to wait.
Because for around $50 (not your $50 of course, but your boss’s or your parents’ – or maybe you pirated it because why pay for software when you can buy Big Macs?) you could finally stick it to your local printer and Hallmark and all those other greedy bastards who picked your pocket and emptied your stomach. Hooray!
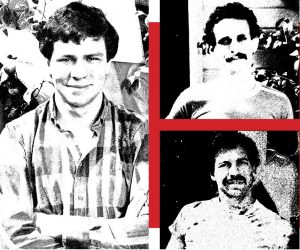
You could print out signs and posters for just about anything, as many as you liked. You could print our greeting cards for friends, family, everyone and anyone – even your dog, cat and goldfish (“Thank you for being such a lovely fish!”) You might even print out a greeting card congratulating you for your newfound ability to print out greeting cards! Why not? All it cost was a bit of ink and paper! But you weren’t the only one ecstatic for the prospect of this new innovation freeing you forever from the joint tyranny of Big Printing and Big Crayon. There were plenty of other people who needed to stretch a buck, and not just so they could stuff themselves with Big Macs. These included not-for-profit organisations who had previously done much of their in-house printing using wood blocks and ink pads (what is this, the 15th century?) and small businesses, which could now use the same computer and printer they bought for doing accounting to print flyers and posters.
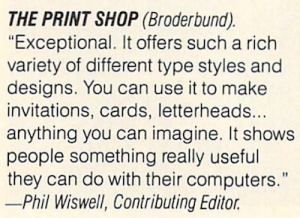
Schools were also really big customers, The Print Shop being a saviour for time- and cash-poor teachers traditionally forced to rope students into drawing banners and posters – not always the easiest of tasks, and one that required some measure of supervision lest little Jimmy stick the felt markers up his nose. To sweeten the deal, Brøderbund came out with an additional ‘companion’ disk that could print customised calendars – what 1980s teacher wouldn’t want that? – and more fonts and graphics. It was an easy sale to make.
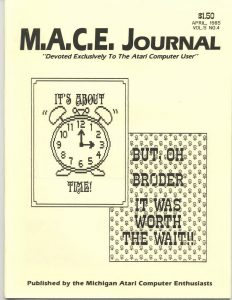
And boy did they sell! Sales took off like a rocket and developers Martin Kahn and David Balsam were rich overnight. The teenage whiz kid Brøderbund CEO Doug Carlston found to convert the program to work on other computers very quickly owned a Porsche! They no longer needed to worry about saving enough pocket change to buy a Big Mac (lucky bastards!) But The Print Shop did much more than simply enable its creators to purchase Porsches full of Big Macs – it proved there was a market for its sort of software.
With no self-interest at all, the publishing industry was extremely skeptical of the emerging threat posed to them by low-cost home computers and dot-matrix printers, mocking the ‘poor quality’ output they produced as unsuitable for anything anyone would dare show to anyone else – but The Print Shop demonstrated people’s standards were much lower than they had anticipated. All kidding aside, a new middle ground was established (somewhere between markers and markup) and other software publishers eagerly jumped into it, creating not just ripoffs like PrintMaster, but new software such as The Newsroom, Create-A-Calendar and Awardware, each designed to provide a new ‘good enough’ solution in an area previously limited to professional or handmade alternatives.
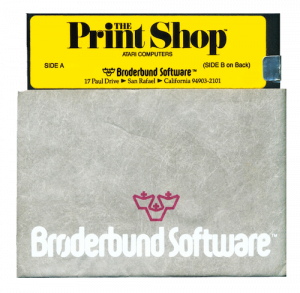
These applications formed the foundation upon which the desktop publishing industry was built –sure, the Macintosh and LaserWriter invaded the commercial printing industry, but they would have had a harder time had software like The Print Shop not aided in fostering an acceptance of computer-generated print media by the general public first. And the demand for consumer self-publishing solutions didn’t end there – lower cost 16-bit computers such as the Atari ST combined with cheaper design packages such as Timeworks Desktop Publisher and higher-quality 24-pin printers meant that those computer users who had previously found such great utility in The Print Shop and its brethren were able to advance in their amateur printing wizardry to full-scale in-the-box design, creating everything from brochures to how-to books in the comfort of their own homes – and without sacrificing too many Big Macs.
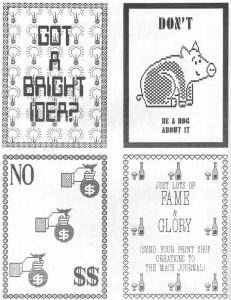
This trend would continue to the present day where obtaining the tools needed to create a publication such as this magazine are within the reach of most. But sadly, while the future was made of happy fonts and clip-art celebrations for many, for some – including Brøderbund’s founders and many of its employees – it would not go so well. After a competitor to The Print Shop called PrintMaster appeared, Brøderbund sued – and won, but this story does not end there, with PrintMaster’s publisher Mindscape making the required changes to avoid legal reprecussions and continuing to market it. But venture capitalist-backed SoftKey, looking to ‘consolidate’ the software industry, saw an opportunity to weaken Brøderbund, buying Mindscape and virtually giving away PrintMaster, hurting Brøderbund’s profits and lowering its stock price. SoftKey mounted a hostile takeover and 500 employees lost their jobs. Bummer!
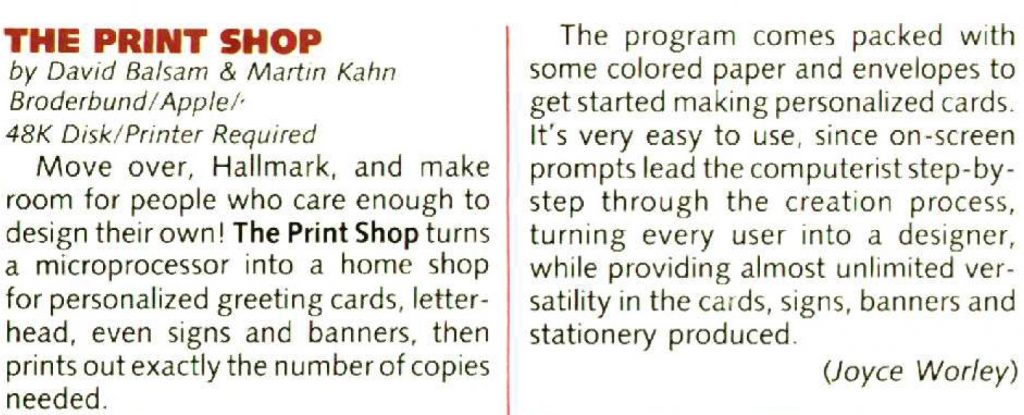